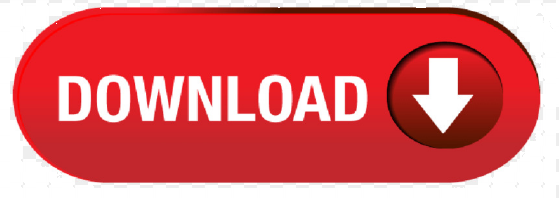
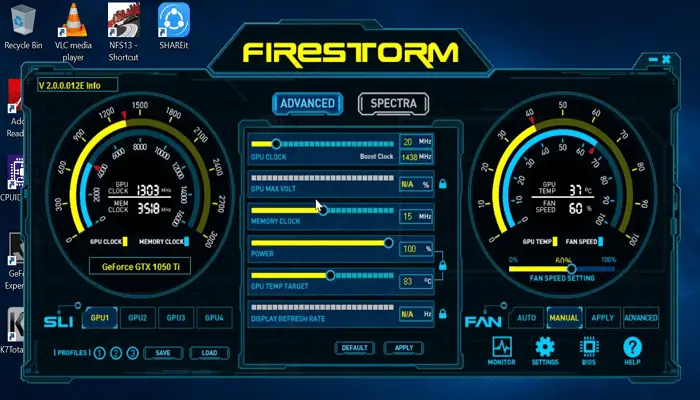
We demonstrate our approach in simulation on problems including car trajectory tracking, nonlinear control with obstacle avoidance, satellite rendezvous with safety constraints, and flight control with a learned ground effect model. We take inspiration from robust convex optimization and Lyapunov theory to define robust control Lyapunov barrier functions that generalize despite model uncertainty. We develop a model-based learning approach to synthesize robust feedback controllers with safety and stability guarantees. %X Safety and stability are common requirements for robotic control systems however, designing safe, stable controllers remains difficult for nonlinear and uncertain models. %C Proceedings of Machine Learning Research %B Proceedings of the 5th Conference on Robot Learning %T Safe Nonlinear Control Using Robust Neural Lyapunov-Barrier Functions
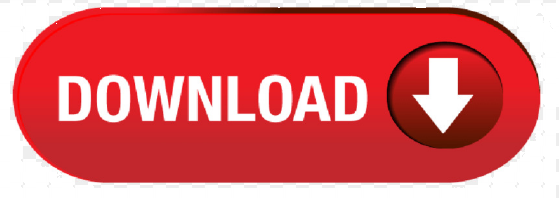